Social Behavior
Effectively modeling and quantifying social behavior
Studying the neural mechanisms of social behaviors requires effectively modeling and quantifying social behavior. Modeling behavior during social interactions remains a significant challenge: the same social behavior may have varied patterns, while distinct social behaviors may exhibit identical frame-level information. Although manual annotation of social behavior can be used to provide a quantitative benchmark for classification, this characterization is biased by the quality of the manual labels, and the results cannot be used to further subdivide the behaviors into interpretable data-driven motifs.
In recent years, data-driven unsupervised techniques have gained traction to circumvent these problems. The strength of the data-driven approach is that the models observe the entire dataset and capture the observed patterns, rather than requiring the user to define behaviors. Our previous work CS-VAE[1], has the ability to model behavioral frames that have continuously varying backgrounds, which is particularly useful in modeling freely-moving socially interacting animals. However, this method fail in automatically picking out different social behavioral motifs.
In our recent work, we model social behavior as a hierarchical set of motifs, we embed high-dimensional behavioral images into a lower-dimensional, informative space using a transformer-based autoencoder[2]. We take a semi-supervised approach to add interpretability by simultaneously decoding the annotated social behavior and reconstructing the behavioral video. By constraining part of the latent space using a Cauchy-Schwarz divergence, we successfully disentangle the latent space into position-based vs. social latent variables. This model facilitates the unbiased quantification of social behavior and can be applied in future studies to reveal the neural mechanisms of social behavior.
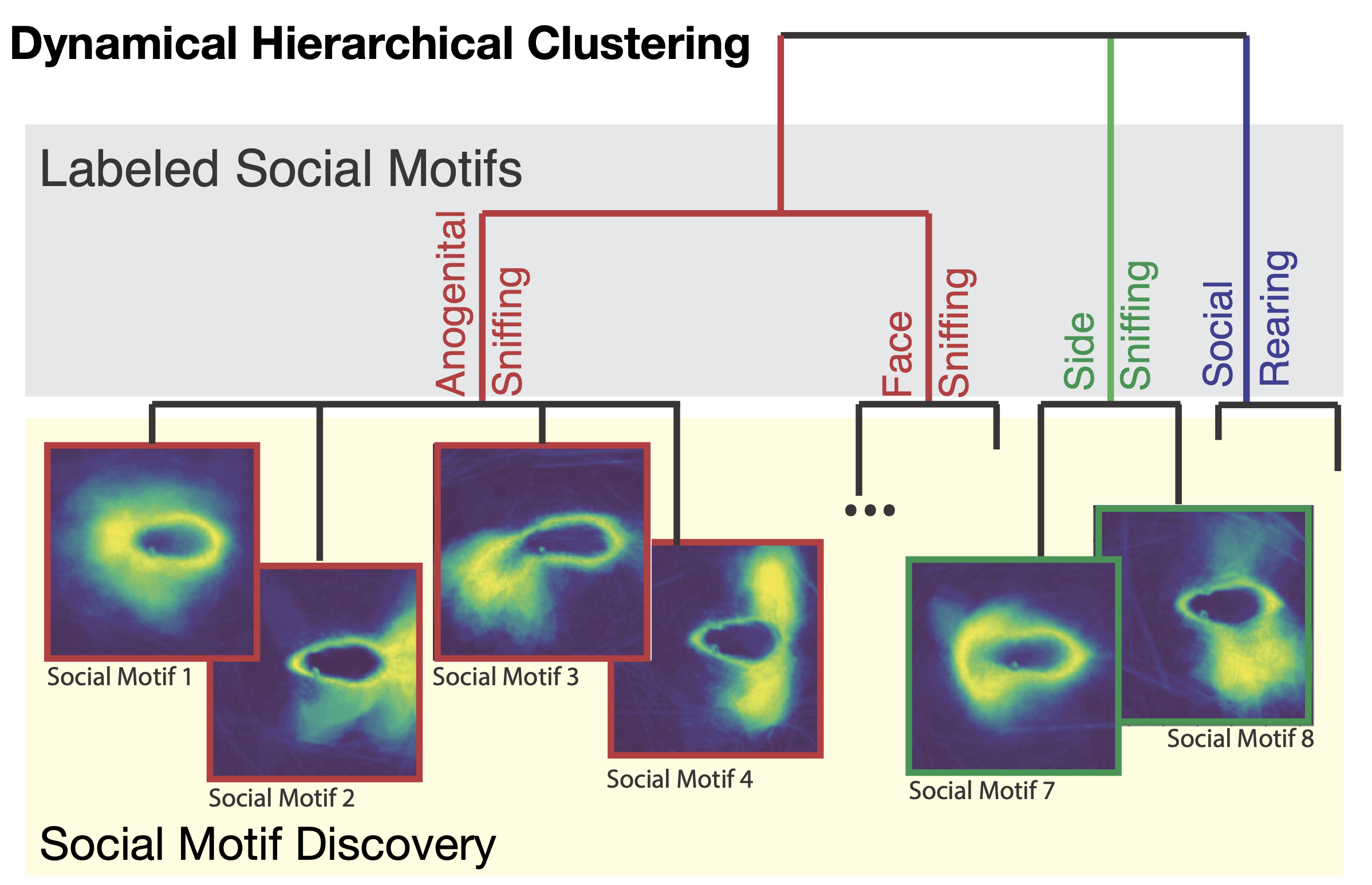
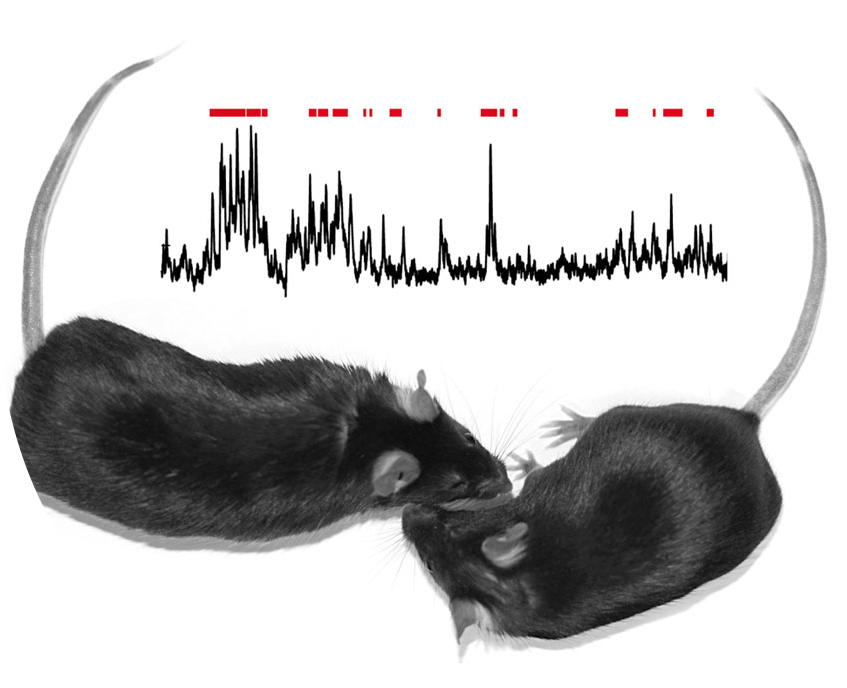
[1] Yi Daiyao, Musall Simon, Churchland Anne, Padilla-Coreano Nancy, Saxena Shreya (2023) Disentangled multi-subject and social behavioral representations through a constrained subspace variational autoencoder (CS-VAE) eLife 12:RP88602
[2] Yi, D., Wright, E., Padilla-Coreano, N., Saxena, S., “Hierarchical Characterization of Social Behavior Motifs using Semi-Supervised Autoencoders”, available @ MABe2023